What Is Deep Learning? How Does It Work?
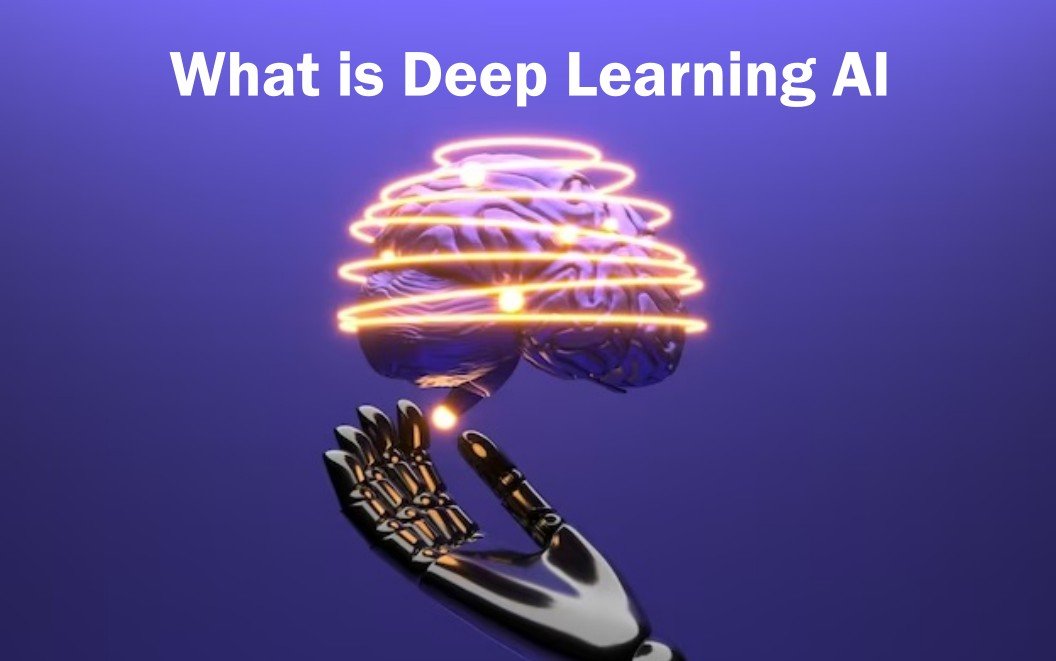
Credit - Freepik
Deep learning is AI-equipped technology that can accurately anticipate future events. It analyzes enormous datasets and classifies them significantly faster than humans. It can work without supervision and give more precise results than machine learning. Due to these qualities, it comes into use in many applications, including text generation and industrial automation.
Deep learning is a significant part of machine learning (ML) and artificial intelligence (AI). It teaches computers how to process data in a way inspired by the human brain. Deep learning models can identify complex patterns in images, text, sounds, and other data to generate precise insights and predictions. It also helps automate processes that need human intellect, such as speech recognition and voice transcription. Here is a brief overview of deep learning AI and its working.
Importance of deep learning
Deep learning is the branch of machine learning that uses neural network models to address complicated problems. These models have features comparable to the structure and function of the human brain. It uses neural networks made of numerous layers of software nodes, similar to how neurons in the human brain connect to learn information.
Deep learning models require a massive amount of labeled data and neural network architectures for training. They can do complex computations at unprecedented speeds, far exceeding human capabilities. Let us know the potential benefits associated with this AI technology.
Also Read -
Autonomous learning capacity
Deep learning systems have an incredible ability to learn and recognize complex patterns. It can evaluate vast amounts of data and automatically extract meaningful features without supervision for adding new features. This quality makes it highly effective in various applications, including natural language processing and self-driving cars.
Volatile data processing
Deep learning systems can categorize and sort enormous data sets via neural network models. With labeled data and computing power, it can work in many areas. For instance, it can analyze complex patterns for fraud detection, digital assistants, and facial recognition. They can offer insights more valuable than other machine learning processes provide.
Unmatched accuracy
Associated algorithms have shown great accuracy across a wide range of tasks. They can outperform humans in voice recognition, object detection, medical diagnosis, and many other projects. Deep Learning systems can attain high precision and low error rates by continuously upgrading their models with authentic datasets.
Flexibility and generalization
Deep Learning models can adapt and generalize new datasets. They can form links within data and make them more adaptable to settings. This adaptability and versatility enables deep learning algorithms to handle complicated real-world issues. They predict accurately even when presented with unseen inputs.
How does deep learning AI work?
Deep learning methods have many layers of interconnected nodes, with each layer set to optimize predictions and classifications. The process causes nonlinear transformations to its input and creates a statistical model as an output based on its learning. Processing continues until the outcome reaches an acceptable level of accuracy. The term "deep" signifies multiple processing layers to represent data.
Deep learning is statistically different from traditional ML processes because it doesn't require supervision. In conventional machine learning, the programmer provides data and supervises it till the end. As a result, the computing success rate is highly dependent on the programmer's ability to provide accurate data. On the contrary, deep learning autonomously builds the feature without human intervention. The deep learning project passes through the following phases:
- Deep learning systems need substantial data and processing power to return accurate results. So, programmers seek massive data sets and cloud computing in the initial phase.
- With sources, artificial neural networks start processing and classifying data. The responses to a series of binary true or false questions involve intricate mathematical calculations.
- Continuous data processing creates complex statistical models. With time, the deep learning model trains itself and increases the chances of getting accurate results.
To understand the working of deep learning, let us take the example of a facial recognition algorithm. It begins by learning to detect facial edges and lines, then progresses to more significant facial features. Eventually, it recognizes the overall representations of faces with time.
Examples of deep learning
Since deep learning models process like a human brain, people can use it for many tasks. Today, programmers incorporate it in natural language processing (NLP), speech recognition software, and image recognition tools. It also has scope in all big data analytics applications. The following are some of the current applications of deep learning:
Self-driving cars
Deep learning AI is one of the trending technologies that facilitate autonomous driving. It can determine whether other vehicles, debris, or humans are around and adjust accordingly. It trains a four-wheeler to drive significantly more skillfully than a human driver could.
Chatbots
Deep learning models can learn everything from data based on human-to-human conversations. These models are now available as chatbots and Chat-GPT. As AI-driven tools continue to evolve, you can expect deep learning in various business applications for better customer service.
Industrial automation
Deep learning systems can help resolve bottlenecks and optimize the supply chain in manufacturing industries. Besides automating production processes, it could enhance worker safety in factories and warehouses. The technology can detect when an employee or object comes too close to a machine.
Facial detection
Deep learning algorithms provide computers with accurate results for facial recognition. It runs through a cascade of multiple levels of processing units for feature extraction. Regardless of cosmetic changes like hairstyles, beards, or poor lighting, it facilitates deep facial identification.
Speech recognition
The CNN-LSTM deep learning models have revolutionized the field of computer vision. These neural networks learn and recognize speech by processing millions of audio clips. They can precisely differentiate different tones and identify the voice of a specific person.
Also Read -
FAQs
1. What is the role of deep learning AI in healthcare?
Ans. Deep learning algorithms can precisely predict future events by analyzing massive amounts of datasets. They can classify data significantly faster than humans. Medical professionals can now use these models to automate many routine tasks. For example, they can use them to manage patient data and identify healthcare trends. They can recommend treatments based on deep-learning results. Technology can help healthcare providers in making better decisions and lowering medical risks.
2. What is the difference between deep learning and machine learning?
Ans. Deep learning is a vital subset of machine learning. The machine-learning workflow involves the manual extraction of pertinent features from data. The ML model processes the available data and produces results accordingly. Contrarily, a deep learning system automatically extracts relevant features from datasets. It undergoes end-to-end learning to achieve accurate results without human supervision.